Water quality AI
The integration of AI and sensor networks for monitoring and improving water quality is a rapidly growing field. By combining real-time data collection with advanced analytics, AI can help optimize water management, ensure safety, and predict future trends. Here’s how AI and sensor networks are used in water quality management:
1.Sensor Networks for Water Quality Monitoring
Sensor networks consist of interconnected devices that measure various water quality parameters. These sensors are often deployed in rivers, lakes, reservoirs, and distribution systems to monitor key indicators such as:
- pH levels
- Turbidity (water clarity)
- Dissolved oxygen
- Temperature
- Conductivity (to measure salinity)
- Presence of contaminants (like heavy metals, nitrates, phosphates, or microbial pathogens)
2.Role of AI in Water Quality Analysis
AI enhances the value of sensor networks by analyzing the vast amounts of data they generate. Here are the key ways AI can contribute:
- Real-Time Data Processing and Anomaly Detection:
- AI algorithms can process sensor data in real-time to detect anomalies or deviations from normal water quality levels.
- Machine learning models can be trained to recognize patterns associated with contamination events, such as chemical spills, and alert authorities immediately.
- Predictive Modeling:
- AI can predict future water quality conditions based on historical data and real-time inputs from sensors. For example, predictive models can forecast algae blooms, contamination, or water shortages, allowing for proactive management.
- AI can also model the impact of environmental changes (like rainfall, industrial discharge, or agricultural runoff) on water quality.
- Optimization of Water Treatment:
- AI can recommend adjustments to water treatment processes based on the data collected from sensors. By continuously monitoring the water quality, AI can optimize chemical dosing, filtration, and disinfection, ensuring safe drinking water while minimizing waste and cost.
- Automated Decision-Making:
- AI systems can be integrated with automated water management systems to take corrective actions without human intervention. For instance, if a sensor detects high levels of contaminants, AI could trigger the isolation of affected areas or adjust treatment protocols immediately.
3.Machine Learning and Data Analytics for Water Quality
- Supervised learning models can be trained on historical water quality datasets to predict pollution sources and their effects. For example, AI could determine that certain pollutants are linked to specific industrial activities or agricultural practices.
- Unsupervised learning models can help identify patterns or clusters in large datasets, providing insights into water quality trends or previously unknown relationships between factors.
- Deep learning algorithms can be employed for image and video analysis, for example, by analyzing satellite imagery to track water quality in large bodies of water (lakes, oceans) or detect changes over time.
4.IoT and Cloud Integration
- Internet of Things (IoT) devices can integrate water quality sensors with cloud-based AI platforms, allowing for real-time monitoring and data processing. The data collected from these devices is transmitted wirelessly to a central database, where AI can analyze it and offer actionable insights.
- Cloud computing allows for scalable data storage and the deployment of AI models across multiple locations, providing regional or global water quality assessments.
5.Applications of AI and Sensor Networks in Water Quality
- Urban Water Systems:
- AI and sensor networks can be used to continuously monitor the quality of drinking water in municipal systems, ensuring it meets regulatory standards and detecting issues like pipe corrosion, contamination, or leaks.
- Agriculture:
- AI-driven systems help monitor irrigation water quality, ensuring crops receive clean water while avoiding the overuse of chemical treatments. They can also track runoff from fields, preventing harmful pollutants from reaching water bodies.
- Environmental Conservation:
- AI models are used to track pollutants in rivers, lakes, and oceans, helping conservation efforts by identifying the sources of pollution and determining the effects of human activities on ecosystems.
- Disaster Management:
- In the event of a natural disaster, such as floods, AI can help predict contamination risks (like sewer overflows or chemical spills) and guide rapid responses to safeguard water supplies.
6.Challenges and Considerations
- Data accuracy and reliability: Sensors can be affected by environmental conditions, and AI models rely heavily on high-quality data. Proper calibration and maintenance of sensors are crucial.
- Data integration and interoperability: With diverse sensors and systems, standardization and compatibility are essential to integrate data across platforms for meaningful AI analysis.
- Cost: While sensor networks and AI offer powerful tools for water quality monitoring, the initial cost of deployment and maintenance can be high, especially for large-scale systems.
- Ethics and data privacy: As more data is collected, ensuring the responsible use of information and protecting data privacy become key concerns.
7.Future Outlook
The combination of AI, sensor networks, and IoT in water quality management is expected to grow as technology advances. AI’s ability to continuously learn from data means that future systems will become even more accurate in predicting water quality issues, ensuring public health, and protecting the environment.
Conclusion
AI and sensor networks create a powerful framework for efficient, real-time water quality monitoring and management. They allow stakeholders—from governments to industries and environmental groups—to make informed, data-driven decisions that ensure the safety and sustainability of water resources.
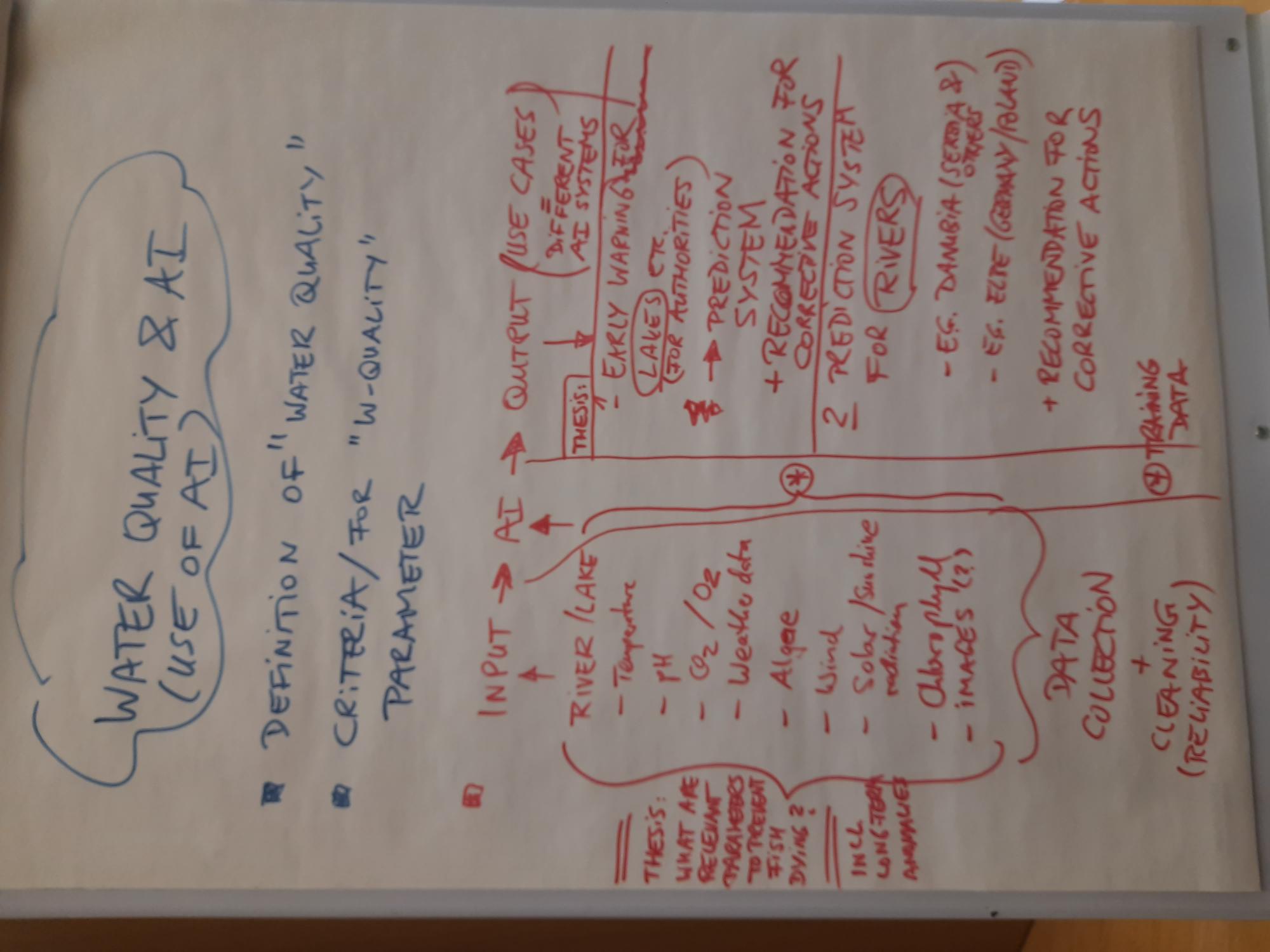
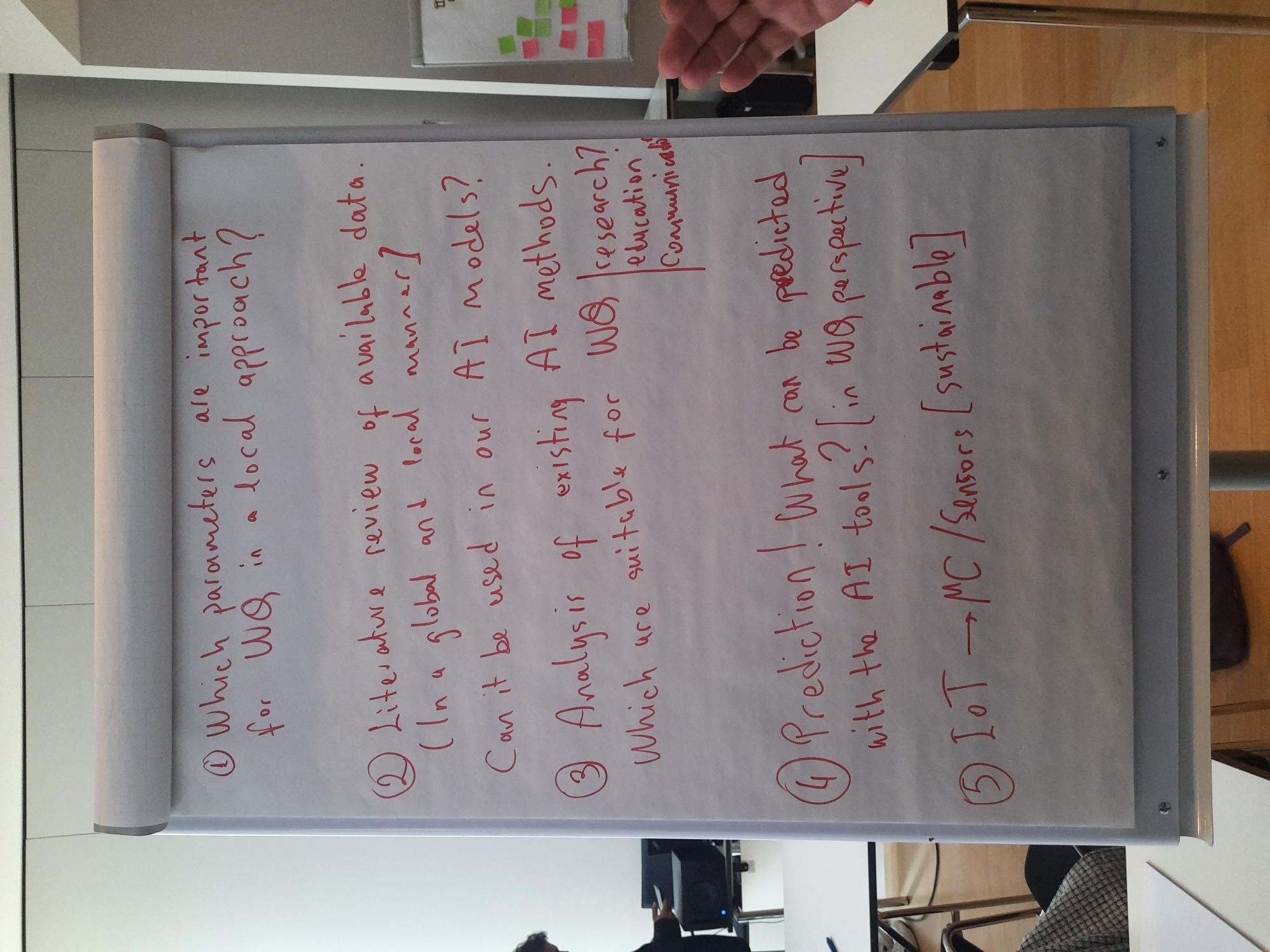
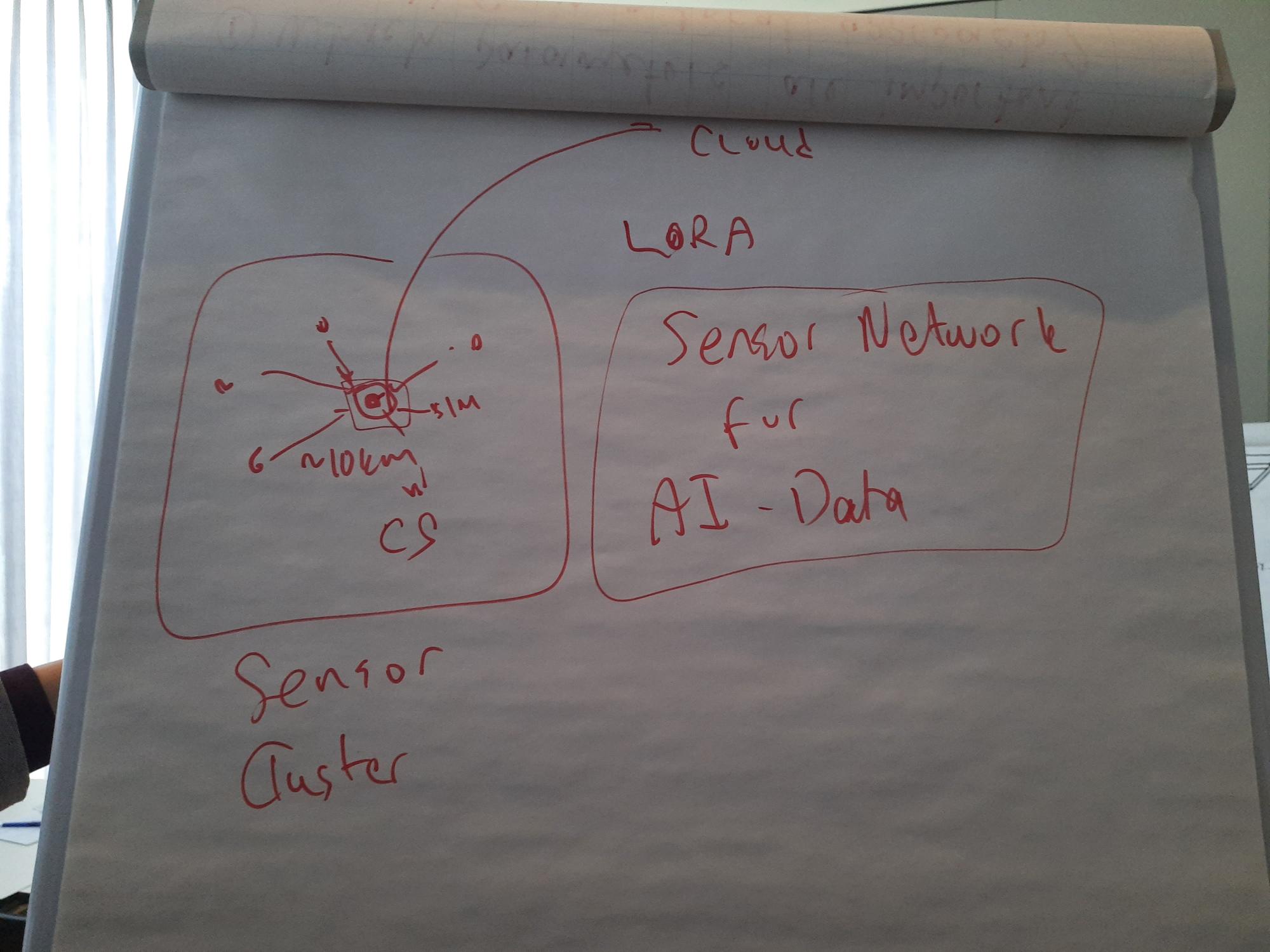